Invest in quality conversations, not wide-ranging chats: closed vs. open generative AI
Subscriber Benefit
As a subscriber you can listen to articles at work, in the car, or while you work out. Subscribe Now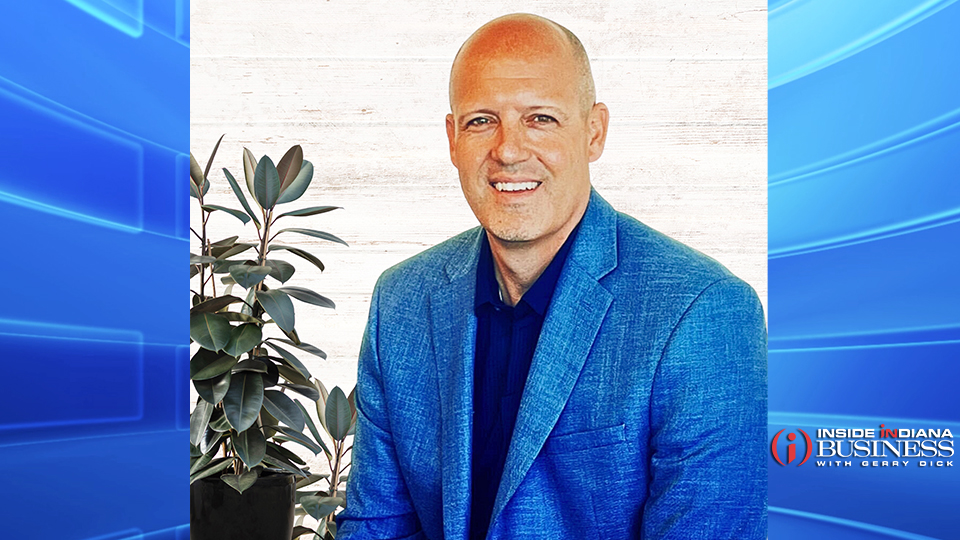
If you’ve ever had a customer service issue and sought a quick resolution, it’s likely that you have interacted with a chatbot. Fast responses, 24/7 availability and scalability have propelled chatbots to be the first line of customer service across a variety of industries. They often perform their job well – a majority of consumers report neutral or positive experiences with chatbots, particularly when looking for a fast result from a simple query.
This efficiency can come with a disadvantage, however: frustration. Chatbots trained on limited data cannot always respond appropriately because they are programmed to respond to specific types of user inputs. According to a report by UJET, 80% of consumers said using chatbots increased their frustration levels and 72% thought chatbots were a waste of time. If a user query falls outside the chatbot’s programming, its responses may not be helpful.
This is where conversational AI comes in.
Most of us are familiar with the general concept of conversational AI thanks to the prominence of ChatGPT. Conversational AI uses Large Language Models (LLMs) and trains on data that contain examples of how people would ask questions or make statements about a specific topic. Then, from these examples, conversational AI forms unique answers from a variety of sources rather than generating a pre-programmed and often “canned” answer.
On the opposite end of the spectrum, generative AI results can be far-reaching. LLMs have the ability to process vast amounts of unstructured data and generate human-like responses to text. For example, open-source ChatGPT is the largest LLM to date and trains on the entirety of the internet to process its responses. With nearly unlimited sources, conversational AI can generate nearly unlimited responses, but that is not always ideal.
The value of a closed solution.
Closed LLM environments are controlled environments where the LLM is trained on specific input data that is not accessible publicly. In other words, closed LLM environments make use of curated datasets. They are often used in regulated industries like healthcare, finance, and legal services where there is a high need for data privacy and security. The curated data sets provide domain-specific knowledge to the LLM, which enables it to generate more accurate responses within the domain of interest.
Closed LLM environments offer a range of benefits over their open-source counterparts. First, they provide better privacy and data security as they are trained on specific datasets that may not be available publicly. This ensures that sensitive information remains confidential. Secondly, they offer greater specificity because they are often curated for a specific domain, such as healthcare or legal services. This allows the LLM to understand and generate responses that are relevant to the task at hand. Finally, they follow established methodologies and best practices, which lead to improved quality and accuracy.
More than Q&A.
Investing in conversational AI tools can benefit your business well beyond one-off customer service questions. Engaging with an individual helps conversational AI learn a person’s preferences while cross-referencing their customer profile. It can turn a Q&A into a funnel for marketing, sales and operations opportunities. Some examples of how conversational AI can be used for consumer personalization include:
- Assisting businesses so they reach the right people at the right time.
- Clear, consistent brand messaging that delivers a natural, human feel without additional human costs.
- Conversational flows for marketing and operations through preferred customer communications channels.
Conversational AI applications are versatile, and using closed LLM environments are ideal for industries like healthcare, finance, legal services and more. Healthcare professionals use closed LLM environments to develop chatbots that can understand patients’ conditions and offer appropriate advice and guidance. In legal services, LLMs can understand legal texts and research cases, making it easier for lawyers to perform their duties. In finance, LLMs can understand stock market trends and predict market changes.
It is important to remember that closed LLM environments require a unique set of methodologies and best practices. Curating the right data sets, cleaning and preprocessing the data are critical for building a high-quality model. It’s essential to avoid common pitfalls like overfitting or underfitting the model and ensuring that the LLM’s training data is a reflection of the domain-specific language used in the target industry.
Closed LLM environments can be a game-changer in the world of conversational AI and all of its applications. They provide an effective way to train LLMs on specific datasets, ensuring that the responses generated are relevant to the task at hand. By understanding the benefits and best practices of working with closed LLM environments, businesses can harness the power of these models to unlock new opportunities in their industries and improve their conversational AI-based solutions’ quality and accuracy.
Josh Ross is the Co-founder and CEO of KLaunch, a company that automates and operationalizes customer journeys through its powerful conversational AI platform, breaking down the technology barriers between people, process and systems.