Purdue prof creates tool to address sinus surgery fail rate
Subscriber Benefit
As a subscriber you can listen to articles at work, in the car, or while you work out. Subscribe Now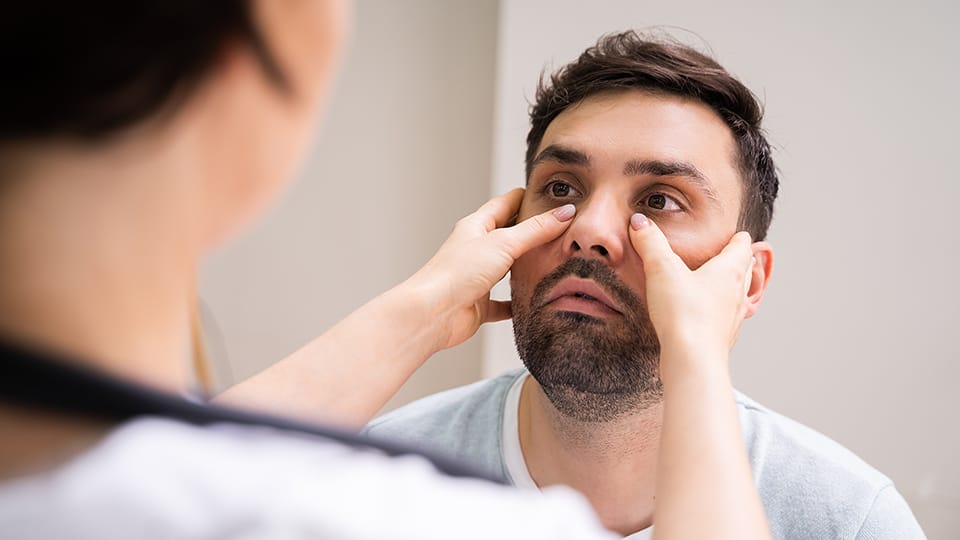
Sinusitis plagues about 30 million U.S. adults, and their doctors often recommend sinus surgery to find relief, but the procedure is notoriously ineffective. A researcher at Purdue University in Indianapolis is sniffing out a solution by creating software—powered by artificial intelligence—that can predict who will and will not have a successful surgical outcome.
Unlike most machine learning, the tool—already being used by some doctors—doesn’t give all the decision-making power to AI, but instead leans heavily on the “human-in-the-loop.”
About 30% to 40% of patients who undergo sinus surgery show no improvement, says Dr. Snehasis Mukhopadhyay, a Purdue computer and information science professor. In addition to the physical and emotional impacts on patients, experts say sinus surgery costs the nation $10 billion in medical waste each year; that climbs to $30 billion if indirect costs such as lost work days are included.
Powered by funding from the National Institutes of Health, Mukhopadhyay and a team of researchers have created a tool that uses “human-in-the-loop” AI, also called interactive machine learning, to predict which patients will benefit from sinus surgery. In the team’s model, a panel of doctors represent the “human-in-the-loop.”
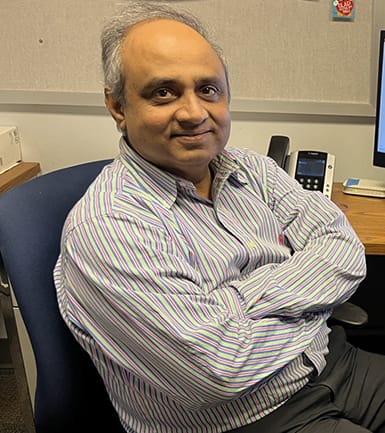
“The machine learning is learning from the data all right, and it’s doing well,” says Mukhopadhyay, “but [we’re asking], ‘Can that be improved any further by leveraging the [human] experts’ opinions?’ So the machine learning and the panel of doctors work in feedback with each other. We showed that improves the machine learning even further. This human-in-the-loop is the really novel approach in our research.”
When used as an advising tool by a doctor, the model achieved 87% percent accuracy in predicting which patients would benefit from surgery, outperforming AI tools that rely on data alone.
“Human beings are not always a set of numbers,” says Mukhopadhyay. “Human beings are multi-dimensional, and there are lots of other variables that come into play [in clinical decision-making].”
The machine learning portion of the prediction tool is analyzing more than 50 variables from each individual patient. The inputs range from clinical measurements such as gender, smoking and tobacco use to socioeconomic data, which includes income, housing status and education level.
Mukhopadhyay says AI is able to make complex connections within data points that humans may not be able to make.
Mukhopadhyay says it’s a critical time to refine how AI is used as it’s becoming increasingly common in the medical industry, from diagnosing patients to remotely treating them. He advocates for leveraging the strengths of both machine learning and humans.
“It’s not either-or. One is relying on data, the other is relying on experience; why not both?” says Mukhopadhyay. “You need to use a combination of human knowledge, experience and intuition with AI. All these clinical and socioeconomic variables [used for AI] may not always be a substitute for the patient-doctor interaction. Machine learning and human doctors should work hand-in-hand. In our case, the machine learning is only a tool the doctors use.”
Mukhopadhyay says translating the model to be used in clinics “is not very difficult at all,” and his medical collaborators at Indiana University School of Medicine are already using it to help decide which patients to recommend for surgery. He believes the tool could reduce unnecessary medical costs and “impact the psychological and physical well-being of individual patients.”
Mukhopadhyay says a drawback of machine learning is its inability to “explain itself,” which often makes humans uneasy.
Mukhopadhyay says keeping the human element in AI will also make it more acceptable to patients. He notes the “black box” nature of AI makes people uneasy, because “you don’t know what’s going on inside; you feed in something, and out comes a decision.”
“This black box AI will not be accepted by people, and AI is here to stay, so how can you bring in the people factor within AI?” says Mukhopadhyay. “I’m convinced this human-in-the-loop approach is the way to go; that’s kind of democratizing AI—making it understandable and accessible to people. Making it more human.”